Privacy and machine learning.
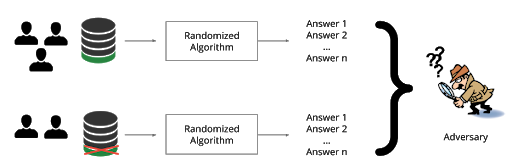
When diving into the world of machine learning, one of the most challenging tasks for an AI company is getting access to large amounts of relevant data, specially in a world as sensitive and private as the banking industry. Banks have long ago understood the value of their data, but they struggle when considering the best way of monetizing it while preserving user´s confidential data. Furthermore, the quality of any machine learning model and AI is limited by the amount and type of data that is trained with, which limits the quality of the predictive models to only the data that each of the entities holds within its systems.
This is why in Fintep, we have adopted a new way of training, maintaining and improving upon our AI: Federated Machine Learning. This approach allows each of the participants in the federated system to contribute and benefit from the training of an AI with the data never leaving their own datacenters, and also minimizing the number of transactions with the outside world, thus reducing possible security threats.
Another advantage that this technique brings into the table is the training speed and market adaptability. Let´s take for instance a problem that a lot of our clients are struggling with right now: LIBOR transition. Given the fact that this has been a relatively recent need in the banking sector, there was no model to deal with this eventuality. Traditional machine learning systems would have required dataset preparation, model training and validation by each of the users separately, which would have resulted in a costly, lengthy task, and with low to medium accuracy depending on the dataset size of each of the entities. But with Federated machine learning, most of this work can be shared without compromising the data, thus extremely accelerating the time to market of functional models as well as increasing significantly the accuracy of the extraction engine.